Introduction
In the ever-evolving landscape of cyber threats, the analysis of password patterns, their vulnerabilities, and the development of enhanced security measures is more crucial than ever. In this article, we delve into an extensive dataset of 820 cracked passwords, all of which were derived from WiFi Access Points across Oslo in 2022.
This insightful journey began with Operation Bloodhound – Cracking WiFi Passwords, where we initially gathered 5800 hashes. After removing duplicates, we honed in on 3962 unique hashes. Of these, 820 were successfully cracked, marking a significant increase in our cracking rate from 13.86% in 2022 to an impressive 20.70% today.
In this piece, we shift our gaze from the technical aspects of cracking and the Open Source Intelligence (OSINT) methods used to identify WiFi owners. Instead, our spotlight is on the cracked passwords themselves. We unravel the patterns, common threads, and vulnerabilities they reveal.
Reflecting on a previous LinkedIn post, the acquisition of a NVIDIA RTX 4090 GPU was a game-changer in boosting my hash-cracking capabilities. This crucial upgrade not only increased my success rate from 18% to 20.70% since that post but also fueled my motivation to write this article. Here, I aim to share an in-depth analysis of the password patterns uncovered in Oslo’s WiFi networks.
Now, I invite you to join me in exploring the world of password analytics.We’ll explore diverse password patterns, common substrings, character types, and frequent passwords, extracting practical insights to enhance password security. Lets dive in!
Distribution of Password Lengths
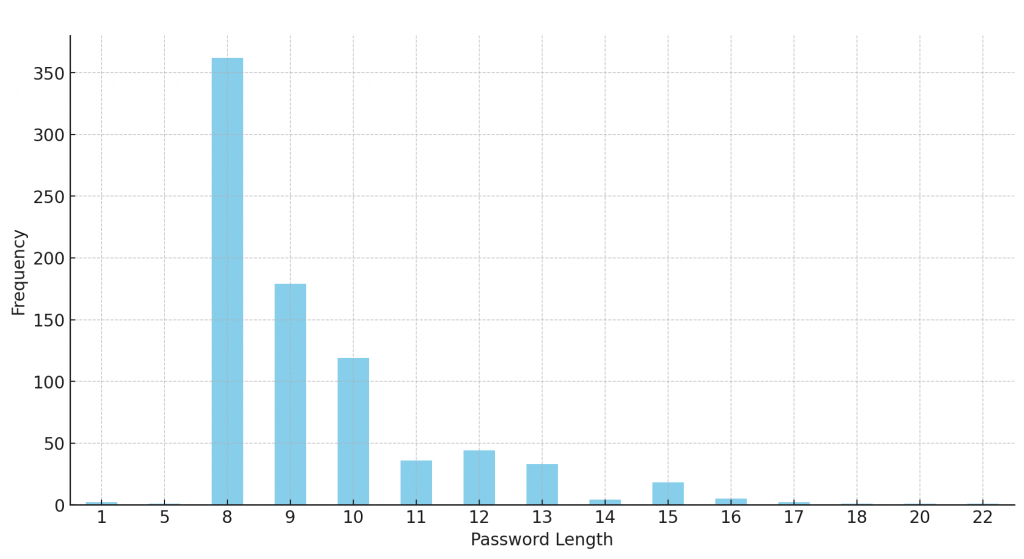
Graph showing the frequency of passwords by length.
The most commonly observed password length in the dataset was 8 characters, accounting for 362 instances, followed by 9 characters (179 instances), and then 10 characters (119 instances). Passwords with lengths exceeding 13 characters were considerably less common.
Password Length | Frequency |
8 | 362 |
9 | 179 |
10 | 119 |
11 | 36 |
12 | 44 |
13 | 33 |
14 | 4 |
15 | 18 |
16 | 5 |
17 | 2 |
18 | 1 |
20 | 1 |
22 | 1 |
Distribution of Password Patterns
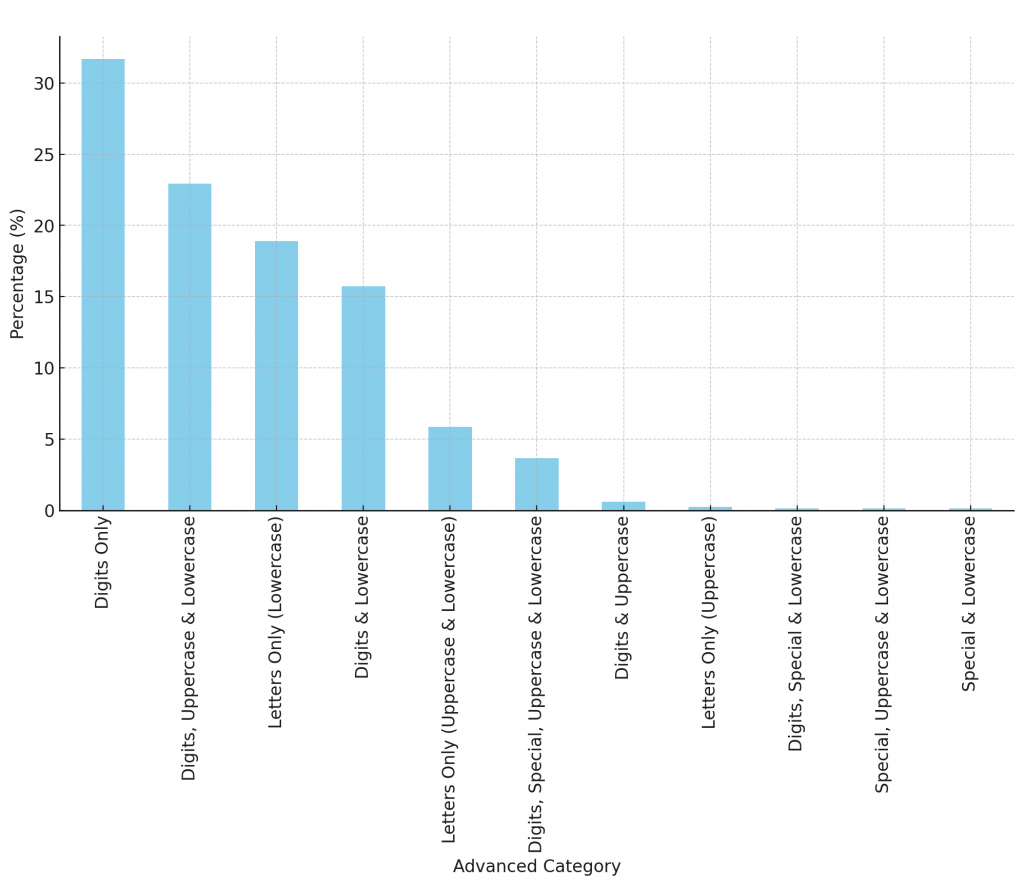
The bar graph presented illustrates the varied composition of advanced password categories as a percentage of the total. The predominant category is ‘Digits Only’, demonstrating a widespread preference for numeric passwords. Following closely are ‘Digits, Uppercase & Lowercase’ and ‘Letters Only (Lowercase)’, showcasing a balance between simplicity and complexity in password choices. Other categories, including ‘Digits & Lowercase’, ‘Letters Only (Uppercase & Lowercase)’, and ‘Digits, Special, Uppercase & Lowercase’, though less prevalent, represent a notable segment of password diversity.
Advanced Category | Percentage (%) |
Digits Only | 31.71 |
Digits, Uppercase & Lowercase | 22.93 |
Letters Only (Lowercase) | 18.90 |
Digits & Lowercase | 15.73 |
Letters Only (Uppercase & Lowercase) | 5.85 |
Digits, Special, Uppercase & Lowercase | 3.66 |
Digits & Uppercase | 0.61 |
Letters Only (Uppercase) | 0.24 |
Digits, Special & Lowercase | 0.12 |
Special, Uppercase & Lowercase | 0.12 |
Special & Lowercase | 0.12 |
This categorization sheds light on the multifaceted nature of password creation. It underscores a pronounced trend towards digit-based passwords, either standalone or blended with alphabetic characters, and a less frequent but still significant use of special characters. Such insights are invaluable for developing robust password policies and enhancing overall security frameworks.
In the dataset, there are no passwords that fall under the ‘Special Characters Only’ category. This indicates that none of the passwords consist exclusively of special characters without any digits or letters. This trend suggests a user preference for including alphanumeric characters in their passwords rather than relying solely on special characters.
Top 10 Common Substrings in Passwords
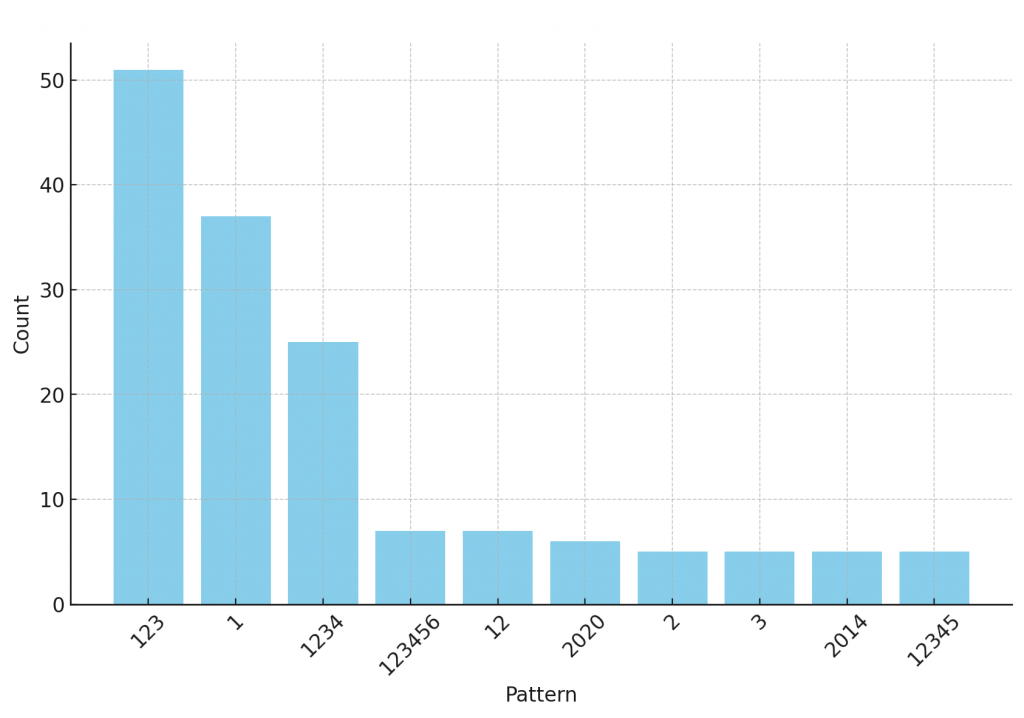
The bar graph above illustrates the “Top 10 Common Substrings in Passwords”. It shows the frequency of various patterns found within a set of passwords. The pattern “123” appears to be the most common, occurring 51 times. Other patterns, such as “1”, “1234”, and “123456”, also show notable frequencies
Pattern | Count |
123 | 51 |
1 | 37 |
1234 | 25 |
123456 | 7 |
12 | 7 |
2020 | 6 |
2 | 5 |
3 | 5 |
2014 | 5 |
12345 | 5 |
Top 10 Most Frequent Passwords
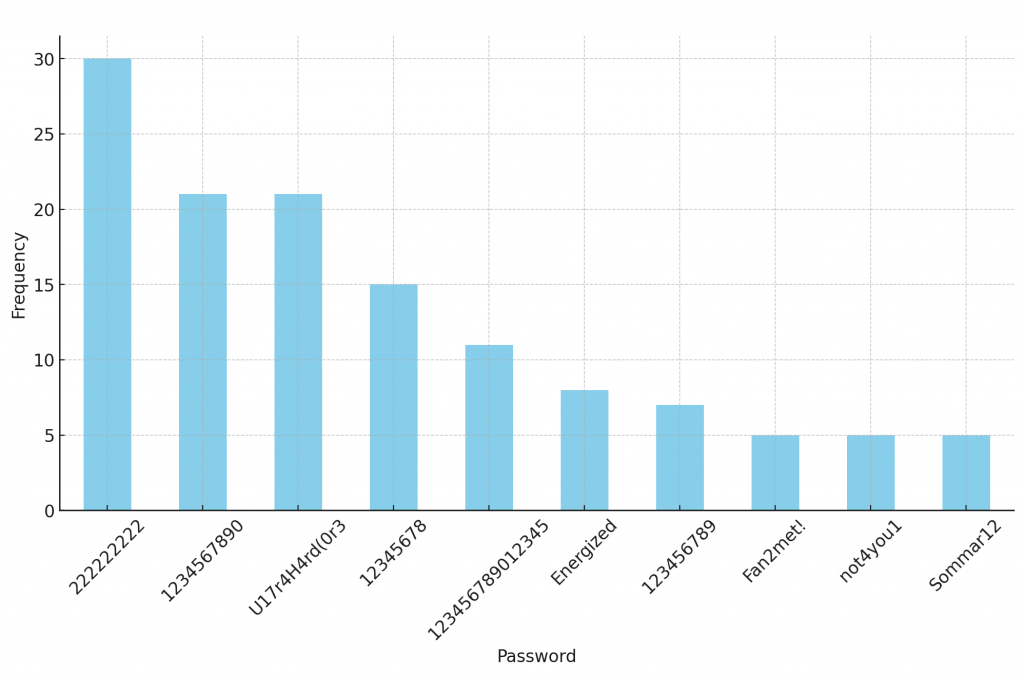
The bar graph above displays the top 10 most frequent passwords in the dataset. It clearly shows that “222222222” is the most common password, followed by “1234567890” and “U17r4H4rd(0r3”, each with significant frequencies. This visualization highlights the popularity of certain passwords, many of which follow simple numeric sequences or common patterns, underscoring potential security concerns in password selection practices.
Password | Frequency |
222222222 | 30 |
1234567890 | 21 |
U17r4H4rd(0r3 | 21 |
12345678 | 15 |
123456789012345 | 11 |
Energized | 8 |
123456789 | 7 |
Fan2met! | 5 |
not4you1 | 5 |
Sommar12 | 5 |
Key Observations and Analysis
Personal Identification: In numerous instances, the Wi-Fi network names (SSIDs) or the passwords were found to be directly linked to personal identifiers such as names, addresses, and phone numbers. This facilitated the easy identification of the network owners.
These examples have been anonymized to protect the privacy of the individuals involved. The original data contained specific personal identifiers such as names, addresses, and phone numbers, which when combined with the network details could potentially be exploited for malicious purposes. As a responsible and ethical entity, we must ensure that privacy is upheld at all times. Therefore, while we discuss these examples to educate about potential risks and encourage safer practices, we have replaced the original identifiers with fictional ones to prevent any potential misuse of the information.
MAC | Password | SSID | Latitude | Longitude | User Notes |
XX:XX:XX:XX:XX:XX | 9999999 | XXXXX | 59.917053530634746 | 10.727484186379696 | Jane Doe 1, Gatename gate 2, 0166 Oslo. Nr: 9999999 |
XX:XX:XX:XX:XX:XX | 13201320 | Navnesen | 59.917053530634746 | 10.727484186379696 | Navn Navnesen, Street name 6 B, 0122 Oslo, Nr: xxx xx xxx |
XX:XX:XX:XX:XX:XX | hyggelig | Jane Cozy Wifi | 59.917053530634746 | 10.727484186379696 | Jane Doe 2, StreetName 6 B, 0252 Oslo, Nr: xxx xx xxx, |
XX:XX:XX:XX:XX:XX | StreetName | 45d | 59.917053530634746 | 10.727484186379696 | Address: StreetName33, 0100 Oslo |
XX:XX:XX:XX:XX:XX | askepott | Jenny & Jane longest reach | 59.917053530634746 | 10.727484186379696 | Jenny Doe, Random Street 22, Nr: xxx xx xxx |
Explanation:
- For the first one nr 1, the password is the user’s phone number, making it easier for someone to guess.
- For “Navnesen”, the SSID is the last name of the user, making them identifiable.
- For “Jane Cozy Wifi “, the SSID is a personalized phrase, which could potentially lead to identification of the owner.
- For SSID “45d”, the SSID was the house number and the password was the street name, making the physical location easily identifiable.
- For “Jenny & Jane longest reach”, the SSID contains the names of the owners, revealing their identities.
Location-Based Identification: Certain networks had their SSID inclusive of an address or apartment number. This, coupled with the geographical coordinates of the Wi-Fi signal, allowed for precise tracking of the network’s physical location and identify the owner of the wifi network faster.
Risks and Vulnerabilities: The simplicity involved in cracking these passwords and identifying network owners reveals major security risks. Personal networks with easily identifiable information can lead to breaches in privacy. Business networks, particularly those belonging to large corporations, pose an attractive target for cyber attacks. The vulnerability of the electric car brand’s Wi-Fi network represents an additional security concern.
Business Networks and Institutional Networks: Networks associated with a diverse range of entities, including a production company, the IoT network of a prominent gym chain, and the administrative network of a notable educational institution in Norway, were included in this analysis. The analysis revealed a trend of using elementary passwords, often just a sequence of numbers or basic words, across these networks. The SSIDs of these networks were frequently identifiable, pointing to a general oversight in network security practices within both the business and educational sectors.
Common Patterns in Passwords: A recurrent theme in the dataset was the use of passwords derived from well-known wordlists such as “rockyou” and “hashmob”. This trend suggests a widespread lack of strong, unique password creation strategies. The concern for network security becomes particularly acute in the context of sensitive networks, like the gym chain’s IoT system and the administrative network of the educational institution. These networks, due to their potentially high-stakes nature, are at a greater risk when relying on easily guessable or common passwords. This pattern was especially pronounced in the case of the gym chain’s IoT network and the educational institution, highlighting a critical need for strengthened password policies in such environments.
Electric Car Brand Network: A popular electric car brand’s service Wi-Fi network was also found to be easily cracked. Tests confirmed that the car would connect to the access point set up by the author, highlighting a potential security issue.
Legacy Software and Equipment: Despite most traffic being encrypted and the presence of firewalls and defender software on computers, there may be legacy software and equipment still in use. This, along with equipment on the network that either has no password or a default password, may be vulnerable to attacks.
Recommendations
- Stronger Password Policies: Both individuals and businesses should adopt stronger, more complex password policies. Passwords should be long, include a mix of character types, and avoid common patterns or identifiable personal information.
- Regular Security Audits: Regularly update and audit network security, especially for businesses, to prevent unauthorized access.
- Education on Network Security: Raise awareness about the importance of network security and the risks of using weak or easily guessable passwords.
- Limit Personal Information in SSIDs: Avoid using personal information in network names to reduce the risk of identification and potential targeted attack.
- Use of Advanced Security Measures: For businesses, especially those handling sensitive data, the implementation of advanced security measures like WPA3, VPNs, and regular monitoring of network activity is recommended.
- Human Error Reduction: Implement password managers in organizational settings to minimize risks associated with password reuse and human error.
- Crafting the Unbreakable WiFi Password: Complexity is Key. Aim for a password that is both lengthy (over 16 characters) and intricate, blending upper and lower case letters, numbers, and symbols. This combination not only makes it challenging for brute force attacks but also ensures the password isn’t easily guessable. Steer clear of using only one to three dictionary words and personal information to further enhance security
- Utilize Passphrases: Consider using a long passphrase – a sequence of words or other text – which can be easier to remember and just as secure as random characters if sufficiently long and complex.